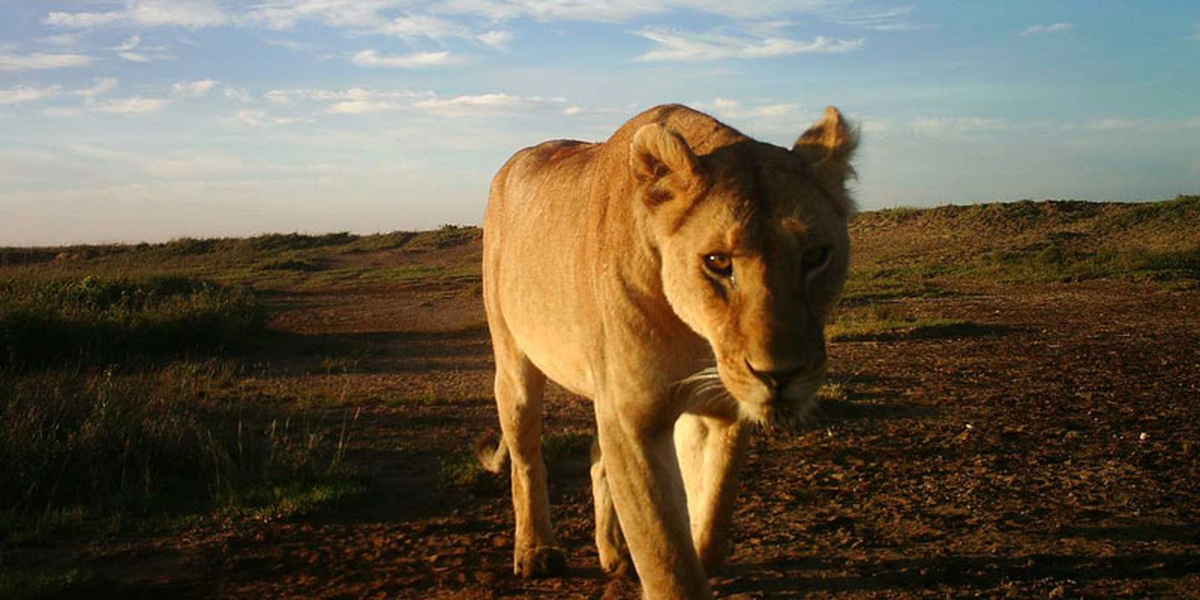
Trail cameras allow conservation biologists and reserve managers to learn how animals move across a landscape. This information is incredibly valuable when it comes to data-informed decision making.
Researchers with the University of Minnesota Lion Center use over 2,000 cameras across different grids in six African countries. These cameras capture over 1.5 million images annually. Even with thousands of volunteers working to identify images through Snapshot Safari, it can take eight to 15 months from when the photo is captured to final classification of the animals in the images.
Increasing the speed and precision of image identification is bound to have significant impacts on work on the ground. Understanding where wildlife are headed is helpful to anticipate potential conflicts between farmers and wildlife. For example, if researchers notice elephants are moving closer to orange groves, they can set up barriers before a conflict occurs.
Volunteers sort through many images that don’t contain any animals. When they find images that do, they identify the animal, count how many are present and note whether an animal is eating or on alert for predators. Artificial intelligence (AI) is poised to drastically expedite this process.
“Conservation biology is a crisis discipline. We’re toeing the line of species going extinct, and the faster managers can respond, the better. We hope to have results within weeks instead of months,” says Sarah Huebner, a Ph.D. candidate in the Packer Lab and research manager of Snapshot Safari.
Seeing this time delay as a major constraint, Craig Packer, director of the center, reached out to collaborators with expertise in AI at the University of Wyoming several years ago. With a large library of classified images, the lab was in a good position to develop, train and test algorithms.
Through this collaboration, the team developed and implemented a tool that identified species well. It was limited, though, as it struggled to count species and identify behaviors.
Huebner reached out to staff at Microsoft working in the AI and conservation space to discuss potential collaborations. In combination with the Lion Center and Zooniverse, Microsoft AI for Earth created an online library to share labeled camera trap images with folks eager to build specialized algorithms to analyze images. The Snapshot team is hard at work developing and testing new algorithms to improve AI recognition of counts, behaviors and demographics of wild animals.
Other AI developers are creating algorithms to detect human activity on camera traps. Managers of protected areas are interested in getting alerts when human activity is reported in areas they wouldn’t expect humans to be. This would allow their team to quickly converge on the spot if poaching is suspected. —Claire Wilson
About the article | This article originally appeared in the Fall 2021 edition of BIO, a magazine for College of Biological Sciences alumni, donors and friends.